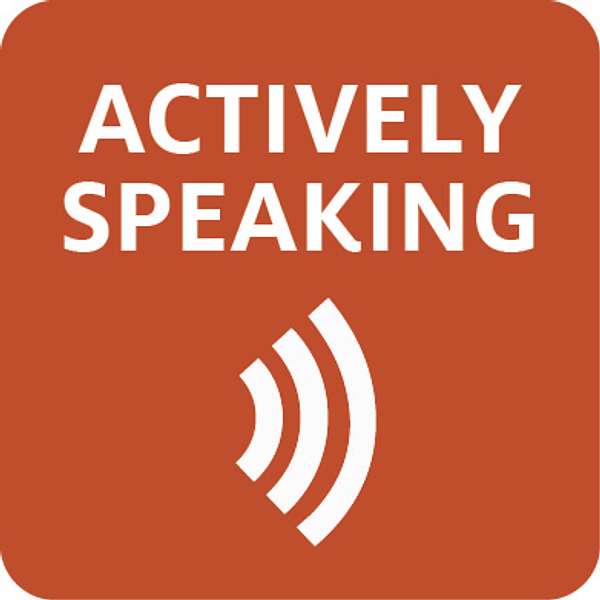
Actively Speaking Podcast
Steve Bleiberg, portfolio manager and thought leader at Epoch Investment Partners, Inc. (TD Epoch) takes on current topics and issues facing today's investor.
Actively Speaking Podcast
Data-Driven Investing: Responding to the Pandemic
In the beginning of 2020, as COVID-19 infections began to surge globally, many investors were confronted by the same challenge, a severe lack of data. Hear from Epoch's Systematic Strategies team on how they've been utilizing data science and in house data sets to visualize the ever changing market environment and make informed portfolio decisions during this crisis. (July 9, 2020)
Important Disclosures:
For institutional investors only. TD Global Investment Solutions represents TD Asset Management Inc. ("TDAM") and Epoch Investment Partners, Inc. ("TD Epoch"). TDAM and TD Epoch are affiliates and wholly owned subsidiaries of The Toronto-Dominion Bank. ®The TD logo and other TD trademarks are the property of The Toronto-Dominion Bank or its subsidiaries. The information contained herein is distributed for informational purposes only and should not be considered investment advice or a recommendation of any particular security, strategy or investment product. The information is distributed with the understanding that the recipient has sufficient knowledge and experience to be able to understand and make their own evaluation of the proposals and services described herein as well as any risks associated with such proposal or services. Nothing in this presentation constitutes legal, tax, or accounting advice. Information contained herein has been obtained from sources believed to be reliable, but not guaranteed. Certain information provided herein is based on third-party sources, and although believed to be accurate, has not been independently verified. Except as otherwise specified herein, TD Epoch is the source of all information contained in this document. TD Epoch assumes no liability for errors and omissions in the information contained herein. TD Epoch believes the information contained herein is accurate as of the date produce...
Hello
Speaker 2:And welcome to Actively Speaking. I'm your host, Steve Weiberg. Join us each episode as we discuss current issues concerning capital markets and portfolio management from the perspective of an active manager.
Speaker 1:Hello everyone. Welcome back to another episode of Actively Speaking. And today, uh, we have not one, not two, but three guests joining me are all from our systematic Strategies team at Epic. Uh, they are Lillian Qua, Lynn Lynn and Simon Chang. So welcome everybody. Hi Steve. Good
Speaker 3:To hear.
Speaker 1:We're gonna talk today about the topic we're calling Data-Driven Investing and the environment. We've been Living Truths the last three, four months with the Covid Pandemic has provided a really good, uh, example of, of how you can use data, innovative uses of data in investing. So I'm gonna start by talking about that, the, uh, the Covid data. But whenever we will broaden the discussion out a little bit later, the, to talk, you know, beyond this environment, how interesting uses of data going forward. So let's start by talking about the pandemic and, and the data. So your team has been making some presentations both internally and externally about what's going on with the pandemic. And interestingly, you've been basing all of this on your analysis on an extensive dataset that you built in-house. So why don't we start by talking about why you chose to do that. Why did you build your own data set rather than simply relying on, you know, some, some well-known data set that's that's out there?
Speaker 3:Thanks, Steve. This is a really good question. Nowadays we have a lot of websites reporting extensive information related to covid, so it's very natural to ask why bother, but this was not the case, uh, before March when this viral outbreak became a pandemic. So like everyone, we began to follow the development of Covid 19 cases in China in January. We very quickly realized that this outbreak could be, uh, very unique and significant challenge for investment. And we were disproportionately exposed to this risk in our ER portfolio where China is 40% of the benchmark weight. So in order to understand this new risk, the biggest problem at that time is actually lack of data. We had to compare with whatever scatters or sometimes contradictory day data point, which news media chose to report. So I remember a few days after the Wuhan lockdown on January 23rd, I found out about a Chinese website called, or dxy, you can see it in the footnote of almost all the prominent global covid tracking platform. Now it is a website created for, um, by healthcare professionals in China and has a great reputation of being honest. And website just started to collate different daily case data in China on the national, provincial and city level. So it's in Chinese and provides only current day updates. So I went to Simon and asked if there's a way to scrape the webpage and put together a hard series data set going forward. He took a look at the website and said, sure. So a day later he created the first Tablo dashboard. We have three pages at a time. Now we have a process that automatically brings in data from many different websites and updates, uh, for the 50 pages of global reports on a daily basis.
Speaker 1:Okay. So it sounds like at the very beginning, there really was no data available out there. Uh, as time went on, more and more data did start becoming available. Why did you choose to continue going on with with building your own database
Speaker 3:Essentially? Ah, another great question. Just like cooking, right? Everybody has more or less the same ingredients, but different dishes require different ways to cook. So before covid, our goal has always been from an investment standpoint how to further our understanding of a new and rapidly evolving with event. So first we need a system to efficiently track new developments globally from one or two glances. We want to be able to have a basic understanding of a big picture every day and put it in a appropriate kind of perspective. So for example, if you want to know the latest cases and then how it compare versus recent trends or versus other countries or even versus important country benchmarks such as, uh, China and Italy. So in that case, I really did not see any other data providers out there that can create such a powerful visualization to fit our own purposes. So efficient and effective visualization is really our reason number one and second, uh, by having the data at our full disposal, it really created a tremend flexibility for deeper and more meaningful research. So this year covid has been a permanent subject in our research meetings. So together we have come up with quite a few important questions that help us eventually make investment decisions. So many of these questions require us to combine information from different sources. This is impossible if we don't have our own data sets. And lastly, we believe Covid will leave Mark in investment history and we need this data for further of future research and, and also postmortem analysis to get us better prepared the next time.
Speaker 1:Lillian, maybe you could talk a bit about what were some of the biggest challenges that your team had to overcome in putting this data together? Yeah,
Speaker 4:Sure. You know, I think whenever you're dealing with a rare event like a pandemic, it, it can be overwhelming to sort out what's gonna be materially important versus just, just interesting. In fact, I know a number of quad investors who decided to become amateur epidemiologists and spent a lot of time trying a full thousand spread of the virus. We decided not to do this. You know, for one thing, we don't have specific expertise in, in viral epidemiology. You know, we also recognize early on that this is a pretty difficult task. Mean there are lots of assumptions. You can get a wide range of potential outcomes and it didn't seem like the right place to spend our research energy. To us, the more important task was to quickly come up with a framework and that the framework would tell us, you know, what types of information to collect, how to interpret this information and what specific portfolio actions to take. You know, I talked about this framework in some detail during a quarterly webinar last week. So I'll just keep my comments to the, the process of data collection. I think the duration of the relevant data was really a big part of our research efforts. You know, in, in January and February and even going into March, it ended up being a collective learning process for our team. So while, while we're really fortunate to have data scientist such assign on staff, we also had sector specialists, you know, get a shout out to Jerome Van gis who covers healthcare for our team. Cause he's been particularly helpful. He's, he was on top of the clinical aspects of the virus from the very beginning. He continues to keep us informed about progress on vaccinations and, and some of the more the medical issues surrounding the, the virus and how we're gonna fight it. Now, of course, you know, data curation is always an iterative process, you know, just because we want certain types of information doesn't mean that they're easy to get or they come to the exact form that you want or we're still that they even exist at all. You know, in some we have to triangulate. So we up using different types of data to proxy for what we wanted to track. So really that's a lot of the, you know, the difficulty, you know, on a day-to-day basis behind, um, using data and collecting it in a way that actually informs what we wanna do.
Speaker 1:Simon, what were the challenges on the implementation side in terms of just getting the data in-house and figuring out a way to, to be able to access it and visualize it and so on?
Speaker 5:So from a technical perspective, I think the biggest challenge was not really in building out the process to ingest the disparate data sets or even to visualize it. That was actually very quick and pretty easy to do. I think the difficult part was maintaining some semblance of data quality. It was difficult because during the height of covid we were constantly introducing new data points and producing novel analysis, what felt like every other day. However, data quality is something we recognize pretty early on as important, something that we wanted to maintain and actively think about. And I think you'll be pretty hard pressed to find perfect data anywhere, anywhere in the real world. Those really only exist in academia. And I do think there's sort of an art in dealing with the issues that you find within any data set. So we have tried to adjust our numbers as countries change their definitions, remove cases from their cumulative numbers and just the various little games they play. So obviously there will be issues that we cannot fully deal with. For example, when China changed the definition of what it meant to be covid positive multiple times during the span of a single week. But we have tried to be vigilant in curating our data and build out this effective framework to ensure the quality of our data. And that's something that we do to this day. One of the bigger challenges we face is just ensuring the overall quality of our data.
Speaker 1:Hmm, that's, that's very interesting. Well, Lynn, let's go back to you. I'm really curious, once you had all this, you had overcome all these challenges, you had this data set that you continue to update everyday. Have you found times when you were looking at your own data and finding perhaps a different picture than what was being presented to us in the general media?
Speaker 3:Right. I would say sometimes, sometimes, uh, it does give us a different and maybe more nuance and interpretations of the data. For example, media tend to highlight data day changes and really ignore the volatility or even seasonality in data. So when we're looking at, um, moving averages, that definitely can help us have a clear picture. Media sometimes also want to focus one or two aspects. For example, just the new cases ignore other relevant information like testing. So for example, I remembered in March, um, I think every underestimated and risk of covid, uh, in the EM countries such as India and Brazil. And at that time, just because the cases were low, but they didn't look at the testing capacity, uh, in those countries, which were really poor. But more often I would say our data will help us to validate and assign sometimes higher probability, probability to one versus, uh, the other scenarios. And we definitely feel that we have a much more complete picture based on our own data and and framework. For example, um, when we judge the vulnerability of a country or state related covid, we look at our scorecard, we have a table, uh, it us the latest data and recent trends of not only the new cases, but also testing, uh, healthcare system capacity and et cetera. So we're able to have a more reasonable gas rate about what is actually happening. For example, when we see a surge in cases, how reliable is the case data? We can look at the cumulative testing with this population forehand, and we can also know whether the surge is the result of increasing testing or because of new spread and or whether the, the hospitals have enough capacity to handle and whether the search will translate into higher rate, et cetera. So all this in one table. So I really feel like the benefit is for us to have a higher condition about the scenario analysis.
Speaker 1:Okay. Let's turn the discussion out to how you've actually used all this data to help in making investment decisions. So Lillian, can you talk a bit about any portfolio changes in positioning or individual stocks that you've made based on trends in the data?
Speaker 4:Yeah, sure. The positioning evolve as the data evolves. I mean, that's the simplest way to understand it. And it were, they were really driven by the types of research questions we were asking ourselves in January versus February versus March. Uh, I remember back in, in January, we were primarily concerned with the effect that have on the Chinese economy. It was already clear at that point that this was a local pandemic, but the national one. So, you know, it really was about understanding just how bad it was gonna be. Now by the end of February, we could also, we already see that the infection curve in China had already flattened. And when we looked at realtime measures of, um, Chinese economic activity, you know, they were actually trending up. There was recovery that we could start to, um, see the data. So our expectation of the time was for things to normalize by, you know, beginning of April, perhaps the beginning of May. So that's not enough for actually making a portfolio decision. You know, understanding the macro picture is important, but we have to take the analysis to the next level. So what we did was ask fundamental analyst to run these, you know, we on our portfolio holdings and we found that a number of companies in our portfolio with, you know, high exposure to China actually had more resilient businesses than than the market team to be giving them credit for. And on this basis, we ended up increasing our positions in about dozen companies. That was part of a broader portfolio rebalancing. You know, when we got into March, you know, the situation that changed quite, quite dramatically. It was, you know, now a global pandemic. It was clear that we needed to focus on other countries and as, uh, as Lynn mentioned, you know, we were pretty alarmed by what was happening in places like India and Indonesia, you know, that the number of cases were doubling starving rate, I would say, even though these countries weren't even testing much at the time. In fact, I would say that they're still not testing enough today. Uh, and we could see that, you know, it wouldn't take very long for them to be overwhelmed by a spike in hospitalizations. It's not so much even the hospitalizations like, you know, you know, you're basically looking forward and saying, if you're seeing the surge, they're gonna have to impose lockdown policies. And that is indeed as what happened in in India, you know, by the end of March. So, you know, we proactively trimmed our exposure to these, uh, to countries and then we allocated the proceeds to, um, located in other countries, which, you know, we're basically dealing with the pandemic more successfully. That would include China, Taiwan and career. I should point out that, you know, our positioning March was not just driven by the, the case numbers or the covid analysis that we were doing. We also looked at risk forecast that were coming out of our emerging markets country risk model. And that model was basically flashing red for Brazil and Mexico at prime. So we took our exposures to those countries down in our portfolios as well.
Speaker 1:Part of it is to believe these days living in the world we're in, uh, this will end someday, this whole covid pandemic and we won't need to be focusing so intently on all this pandemic related data, but the use of alternate data sources and innovative uses of data is not gonna go away. And that's, that's something we should talk about at the end here. So what sort of, what sort of alternative data sources are you developing for, for use of investment decisions once we get fastest this episode? And how big a role do you see this kind of data gathering and analysis playing in the future?
Speaker 4:Uh, well in a nutshell lot, you know, I, I think there's no<laugh>, um, that's going be untouched by data and the, the power that needs techniques will bring investing, which is really a very data driven industry. I think impact will be more dramatic. You know, at some point it's gonna be table fixed. So lemme just start with the data, right? So there's an notion of information out there and there's more and more becoming available every year. I think if you look at you research budgets for alternative data, I mean, they have to quadruple in a very period of, I don't see that actually turning, uh, anytime soon. You know, for a firm of our size, our goal is not to, to look at everything, but to curate what's valuable for our investment process. Now this, you know, requires a lot more judgment that you might think, you know, this is not the traditional quantitative research where you try to find things that work everywhere and at all times. This is, this is where you, the curation process becomes really, really critical. And, you know, it's not a bad thing. I think it's actually a way for us to differentiate ourselves to get an edge. It really is about understanding, you know, what's valuable for your process and that's actionable. The three categories of data which, uh, I think are priority for us with the text, you know, so I would say most of the data that's out there is not numbers. It's actually text. So there's quite a bit of untapped out there that we wanna understand. We wanna be able to use a powerful way, quite a few language aim to that point. The other is, I guess for lack of a better term, market dynamics, you know, and, and it really has to do with the, the actions of other investors. So we have lots and lots of research on fundamental, I don't think industry, we spend enough time understanding what other investors and the market are doing, how their position flows and whatnot. So I think that's, that's another area that particular us. And finally, you know, we, yes, we do have a lot of information on, on financial information, on financial metrics for, for companies. But, you know, I don't know if we'd really track them over time in a way that we need to. And I, I think in particular about, uh, for specific companies, right? You have KPIs that, and analysts might call out when they pitch a stock. I would say that, you know, most managers maybe don't track those KPIs as religiously as they, as they should. And that's a way that I think you can add a lot of value because, you know, the information's coming in in real time, it's pretty hard to, to, to go back and say, well, that's not what I really meant when, you know, it's already written down in a piece of paper. So that, that's another set of data that I think will be a high priority for us to, to tackle over the next couple of years. On the machine learning side, you know, it really, there are two things we're trying to get outta these new techniques. One is just the model relationships in a more sophisticated way, particularly relationships, which are, um, the other is to really leverage the adaptability that's built into the systems, right? I think investors just have to be much more adaptable today. And machine learning can help because these systems by nature are, are responsive to new information. So that's a way that you can, you know, almost automatically be a little quicker to react to changes in the market environment. So those would be the, you know, the two areas that I, I would be directing, you know, the research efforts for my team, you know, for the next few years.
Speaker 1:Ok. Simon, you're a, you're a data scientist. What is Epic doing to ensure that we are ready for this, this new landscape that Lilian has just described?
Speaker 5:So the data sets are out there are really quite remarkable. And if there's a data set out there that you want and you can't find it, it's likely that you aren't looking hard enough. And all this data is what people are beginning to look at and it's become an arms race for everyone to keep up and to understand what is out there, how to process it and how to make investment decisions off of it. Uh, there's probably not one data set that exists that is a holy grail of everything, but it's about how we can take these disparate data sets, combine them in a thoughtful manner that will give us answers that we're looking for. And the big question is, now that you have all these huge data sets, how do you begin to efficiently process them and look for signal within them? And right now our team has been working extremely hard on building out this new cloud environment on Microsoft Azure that will allow us to do everything that maybe I talked about. And on our team, Chris Keller has been leading that project and has done a really phenomenal job in getting everything set up. So we're still really in the early stages, but what we have seen so far is quite remarkable. And to really kind of, of make the processing of the data possible, you have, you have really had to have two revolutions happen at the same time, but one is that computing power hardware and software have had to go through a revolution and we've really seen that. And price has also fell off the clip for storage. So right now we can go on the what and buy storage on something like Microsoft Azure. We're very cheap. And then when we're done, we, that's it. We can just turn it off. But right now we're still in the early stages, but we are working actively on it. Okay. Well Simon and Lillian and Lynn, thank you very much for joining me and we'll, we'll definitely wanna check back at the point in the future cuz this all sounds really intriguing and I'm sure our listeners will be curious to, to know how this is progressing over time. So thank you all. Okay. And, uh, we'll talk to you everybody again soon. Thank you very much. Thanks.
Speaker 6:Thank you.
Speaker 2:Remember to subscribe to actively speaking on Apple Podcast, Spotify or Google Play. You can find all of our previous episodes and additional content on our website, www.eipny.com.
Speaker 7:The information contained in this podcast is distributed for informational purposes only and should not be considered investment advice or recommendation of any particular security strategy or investment. Product. Information contained herein has been obtained from sources believed to be reliable but not guaranteed. The information contained in this podcast is accurate as of the date submitted, but is subject to change any performance information. Reference in this podcast represents past performance and is not indicative of future returns. Any projections, targets, or estimates in this podcast are forward-looking statements and are based on epic's research, analysis and assumptions made by Epic. There can be no assurances that such projections, targets or estimates will occur and the actual results may materially be different. Other events which were not taken into account in formulating such projections, targets or estimates may occur and may significantly affect the returned or performance of any accounts and or funds managed by Epic. To the extent this podcast contains information about specific companies or securities, including whether they are profitable or not, they are being provided as a means of illustrating our investment thesis. Past references to specific companies or securities are not a complete list of securities selected for clients and not all securities selected for clients in the past year were profitable.